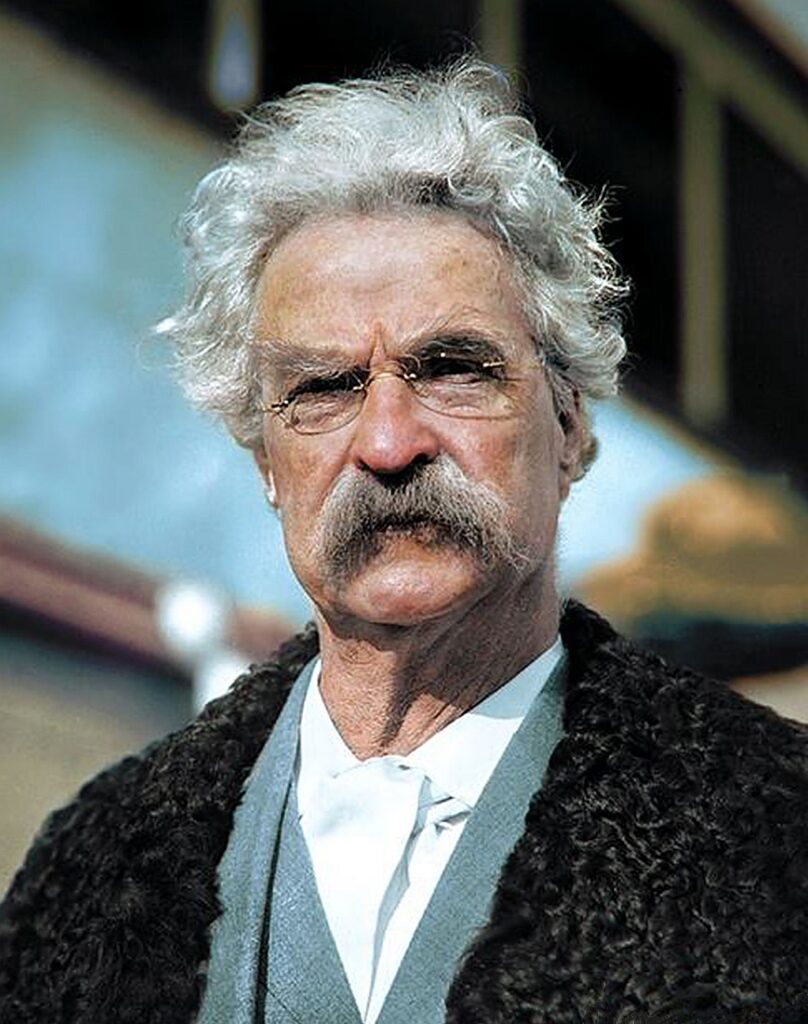
However, I believe it is largely because folks are using subsets of data that may or may not be valid in the long term. Let me explain.
I’ll start by noting that I am not a mathematician. I studied economics in school. However, I’ve always had an innate sense for numbers and stats. When I was young, my twin brother (who is a mathematician) and I played sports games — we even created our own on the Commodore 64 (remember those clunky things?) — and our ultimate goal was always to get the statistics right.
In fact, my brother once wrote to a board game company called APBA about inaccuracies in “APBA Saddle Racing,” a horse racing game that produced absurd race times… and margins… and odds. The response he got makes me chuckle even today: “We found no such inaccuracies.”
I suggested my brother re-write his letter in Braille (this was pre-internet), but he declined.
My point here is not to knock APBA — I still love the game, conceptually — but to point out that I take numbers very seriously. In an increasingly anti-intellectual society, numbers represent the truth. But understanding them and the importance of context is crucial.
One of the biggest issues I see with people who use statistics to build predictive models like me is the reliance on small samples. Many statisticians will tell you that a sample size of 100 is the bare minimum needed to draw any conclusions, but most will generally agree that 1,000 is more than sufficient.
In theory, this makes sense. Flip a fair coin 1,000 times or 100,000 times and the results probably won’t vary much. However, this is only true if everything is “normal.” This is why so many clinical studies are trumpeted as “randomized controlled trials,” or RCTs. We’re all different, so the best way to minimize those differences is to draw from a random pool and separate the participants into an experimental group, i.e. the group getting the substance being tested, and a control group, i.e. the group getting a placebo. The variations between these groups are then observed and analyzed for statistical significance at the end of the trial.
But here’s the rub: What if the groups aren’t normal? For instance, using our previous example of a coin flip, what if we happened to test a group of “skilled” coin flippers and drew conclusions from that?
Think this is absurd? Well, a 2009 study published in the Canadian Medical Association Journal (CMAJ) found that, with minimal coaching, people could flip more heads than tails on command. One study participant was able to “achieve heads 68 percent of the time” — after 300 flips! Imagine if he’s a part of our study group.
Sound far-fetched? Yeah, it is, but it happens every day in different, more subtle ways.
Take, for example, the case of Long-Term Capital Management. LTCM was a hedge fund comprised of financial luminaries and celebrated economists, including two Nobel Prize winners (Robert Merton and Myron Scholes). At one time, LTCM was producing a 40 percent annual return and had assets over $100 billion (about five percent of the total global fixed-income market). Yet, Long-Term Capital Management failed spectacularly — and many speculated it was due to the fund’s computer models, which were based on economic conditions that had subsequently changed.
Ditto Tiger Management.
Run by Julian Robertson, known as the “Father of Hedge Funds” and the “Wizard of Wall Street,” Tiger Management turned an initial $8 million stake into $2 billion in profits over 18 years. Then came the dot-com era.
Robertson, who had built his reputation on buying underpriced stocks and selling overpriced ones (by conventional metrics), was ill-equipped to handle this new, less rational atmosphere, which he admitted in a letter to investors in 2000.
“As you have heard me say on many occasions, the key to Tiger’s success over the years has been a steady commitment to buying the best stocks and shorting the worst. In a rational environment, this strategy functions well. But in an irrational market, where earnings and price considerations take a back seat to mouse clicks and momentum, such logic, as we have learned, does not count for much.”
Tiger Management folded in 2000.
The takeaway is that we don’t know at any point whether our observations and conclusions are based on normal conditions or unusual ones. With a coin flip, we can logically determine the chance of one outcome or another under normal conditions (fair coin, unskilled flipper), making it easier to draw definitive conclusions. If someone flips a coin 100 times and gets 60 tails, we know via binomial probability that the chances of such an occurrence are about 2.8 percent; therefore, we can tentatively conclude that the coin or the coin flipper might not be “normal.”
But how do we do that with the 30-1 shot we like in the fifth race at Santa Anita? Our statistics may tell us the algorithm that produced this horse is profitable, but perhaps those stats are biased by the equivalent of a “skilled coin-flipper” or two in the sample. Maybe they are biased by a preponderance of lower-odds horses, or horses with superior speed figures not considered by the algorithm — the possibilities are endless.
No, the stats aren’t lying, but interpreting them can be challenging.